Remote sensing for Agro-informatics
We develop monitoring systems that can provide globally precise metrics on how cropland is being used. These systems boost various applications (e.g., tree crops mapping and yield predictions) to feed increasing the world population and fight poverty for undeveloped countries.
1. NASA LCLUC: Evaluating land use change and livelihood responses to large investments for high-value agriculture: managing risks in the era of the Green Morocco Plan
This project evaluates the social and environmental consequences of large-scale agricultural investments, focused on (1) the transition from cereals to perennial crops in the drought-prone Mediterranean country of Morocco and (2) the possibility to use remotely-sensed indicators of environmental stress at the basis of responsible, adaptive relief financing.
2. TechnoServe: Mapping Cashew Plantations in Benin
Methods for mapping tree crops or plantations are limited in their spatiotemporal resolution and scalability. Spectral information (e.g. NDVI) alone is often not sufficient to distinguish perennial crops like fruits, nuts and vineyards from other types of vegetation. This project will use variants of deep neural network and very-high-resolution satellite imagery to map cashew plantations (area and counts). Multiple attention-based neural networks will be explored to predict cashew yield and farmers’ practices.
3. Prosper cashew: Map the cashew plantation to help build a thriving and sustainable West African cashew processing industry
The world is consuming more cashews than ever. The global cashew market is a $12 billion industry, and the volume of cashew traded has grown almost seven-fold over the last four decades. While West Africa is responsible for 45% of all raw cashew nut production today, less than 7% of it gets processed where it is harvested. The Prosper Cashew initiative aims to foster a robust and sustainable cashew processing industry within West Africa.
An accurate map of cashew tree distribution constitutes a vital component of this project implementation. It could aid in the strategic positioning of cashew processing plants, thereby facilitating well-informed decision-making that could potentially augment cashew production and processing and mitigate poverty among smallholders. This project will employ domain adversarial neural network and high-resolution satellite imagery to map cashew plantations in West Africa with only a small number of local labels.
4. PepsiCo: Develop mechanistic models and key remote sensing features for Opt-Oat: integrated research of crop modeling, remote sensing, and field zoning
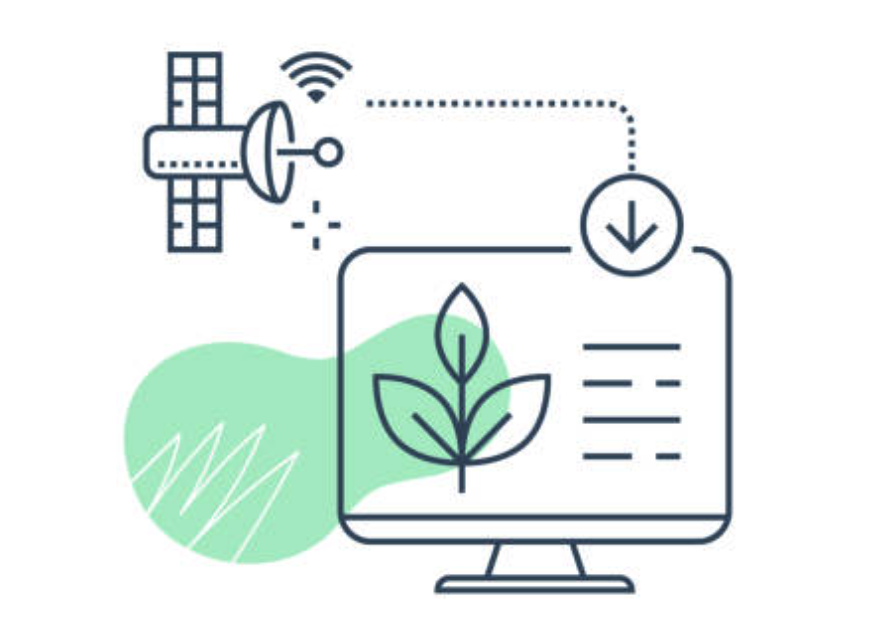
Improving agroecosystem modeling for sustainable management
To maintain food security and environmental sustainability, this research involves process-based modeling and AI-driven methods to track agroecosystem greenhouse gases and biogeochemical cycles of water, C, N and P.
1. NSF SCC-IRG Track 1: Co-Producing Community – An integrated approach to building smart and connected nutrient management communities in the US Corn Belt
Enabling farmers to manage N and P with greater precision is needed to increase farmer profitability and decrease off-farm losses of nutrients, which can compromise water resources. The objective of this project is to develop science-driven recommendations on N and P management that can be tailored to different farmers’ needs, focusing on the heart of the US Corn Belt: Illinois. Through this project, we will: (i) identify major constraints on how Illinois farmers manage N and P; (ii) determine how much N and P are stocked in soils across a diversity of Illinois farms using soil sampling, soil sensors and satellite observations, and how this soil nutrient capital contributes to crop growth in order to model field-specific fertilizer needs; (iii) develop smart and connected technology solutions that enable constrained farmers to join a Nutrient Management Community.
2. NSF SitS: Spatial and Temporal Patterns of Soil N and P Cycles Quantified by a Sensor-Model Fusion Framework: Implications for Sustainable Nutrient Management
High crop productivity in the Midwestern US was achieved by artificially draining wetlands and applying millions of tons of nitrogen (N) and phosphorous (P) fertilizers. However, 40-80% of these N and P nutrient inputs are lost from soils and become pollutants in water bodies and the atmosphere. This project will integrate recent advances in nanotechnology, sensing technology, and machine learning to enable new methods for measuring and managing N and P in croplands to reduce losses to the environment. The outcomes of this project can be used directly by farmers to better manage field application of N and P fertilizers and by local/federal governments and other organizations to pinpoint pollution hotspots and develop strategies for nutrient reduction.
3. DOE ARPA-E SMARTFARM: The “System of Systems” Solutions for Commercial Field-Level Quantification of Soil Organic Carbon and Nitrous Oxide Emission for Scalable Applications (SYMFONI)
Accurate and rapid field-level quantification of carbon intensity at a regional scale is critical to facilitate adoption of new technologies to increase the bioeconomy’s feedstock productivity and reduce its carbon footprint. This project will develop a commercial solution, SYMFONI, to estimate soil organic carbon and the dynamics of nitrous oxide emissions at an individual field level. The solution can be scaled up to perform per-field estimates for an entire region. SYMFONI is a “system of systems” solution that integrates airborne-satellite remote sensing, process-based modeling, deep learning, atmospheric inversion, field-level sensing, and high-performance computing.
4. USAID SIIL Geospatial, Farming Systems, and Digital Tools Consortium: Building a New Era of Predictive Agricultural Innovation to Improve the Livelihood of Smallholder Farmers
This consortium will build upon the five domains — productivity, economics, environment, social and human condition — of the sustainable intensification framework, developing an interdisciplinary and solution-oriented geospatial framework, integrating remote sensing, farming systems modeling, and geospatial data layers to provide innovative data products to take actions toward more resilient farming systems, benefiting families and communities. Providing access to simple digital tools to researchers, extension personnel, policymakers and practitioners will allow them to make informed decisions to minimize risk and improve the resilience of people and farming systems.
5. USDA NIFA: High-resolution integrated assessments of tillage practice impacts on crop production and agroecosystem sustainability in the US Midwest – combining meta-analysis, airborne-satellite sensing, and process-based modeling
Tillage affects production costs, crop yields, and environmental sustainability. This project integrates meta-analysis, airborne-satellite sensing, and process-based modeling to assess tillage’s effects on crop productivity and environmental health in the Midwest, focusing on Illinois, Indiana, Iowa, and Minnesota. We’ll study tillage’s influence on corn and soybean yields and environmental metrics like greenhouse gas emissions and soil erosion. Objectives include: (1) Using historical data for meta-analyses on tillage impacts; (2) Mapping field-scale tillage trends from 2000-2023 using airborne imaging and satellite data; (3) Validating the Ecosys model on tillage impacts using experimental data and satellite measurements; and (4) Assessing tillage’s effects using the Ecosys model to guide policy and farming decisions.
6. NSF III: Medium: Advancing Deep Learning for Inverse Modeling
In earth and environmental sciences, models help predict and understand complex systems like streamflow prediction. These models rely on parameters like slope and soil type, which are often inaccurately known. Inverse modeling estimates these parameters by working backward from observed data. Current inverse modeling techniques are computationally intensive and struggle with large-scale data. This project aims to create advanced machine learning algorithms for inverse modeling that can efficiently handle vast datasets, offering enhanced predictions and reduced computational needs. These innovations can benefit sectors like health, agriculture, and engineering, addressing significant societal issues.
7. NSF CAREER: AI-enabled Integrated Nutrient, Streamflow, and Parcel sImulation for Resilient agroEcosystems (INSPIRE): a framework for climate-smart crop production and cleaner water
Climate-smart agricultural practices hold the promise of reducing carbon (C) emissions from farming, yet their implementation often presents complex trade-offs, particularly affecting nitrogen (N) and phosphorus (P) management. Integrated management of C, N, and P to ensure climate-smart crop production while preserving clean waters is hindered by several knowledge and technology gaps. To approach a solution for this grand challenge, this project aims to significantly advance the holistic understanding and modeling of the interconnected C, N, P, and water cycles in the Upper Mississippi River Basin. This goal will be pursued by developing an AI-based framework of integrated nutrient, streamflow, and parcel simulation for resilient agroecosystems (INSPIRE) that can easily ingest multi-source observations and provide an accurate and speedy quantification from the field to basin scale. The outcomes from this project are expected to provide valuable insights for policymakers and farming communities, particularly in optimizing management practices for improved carbon sequestration, soil health, and water quality in the America’s heartland. Additionally, this project intertwines its research objectives with an educational agenda, which is featured by developing a computational tool to foster broad participations in large-scale computing among undergraduates. The project will also introduce a cyber-physical watershed mesocosm as an innovative trial of using the digital twin technology to enhance STEM education related to agricultural and environmental sustainability.
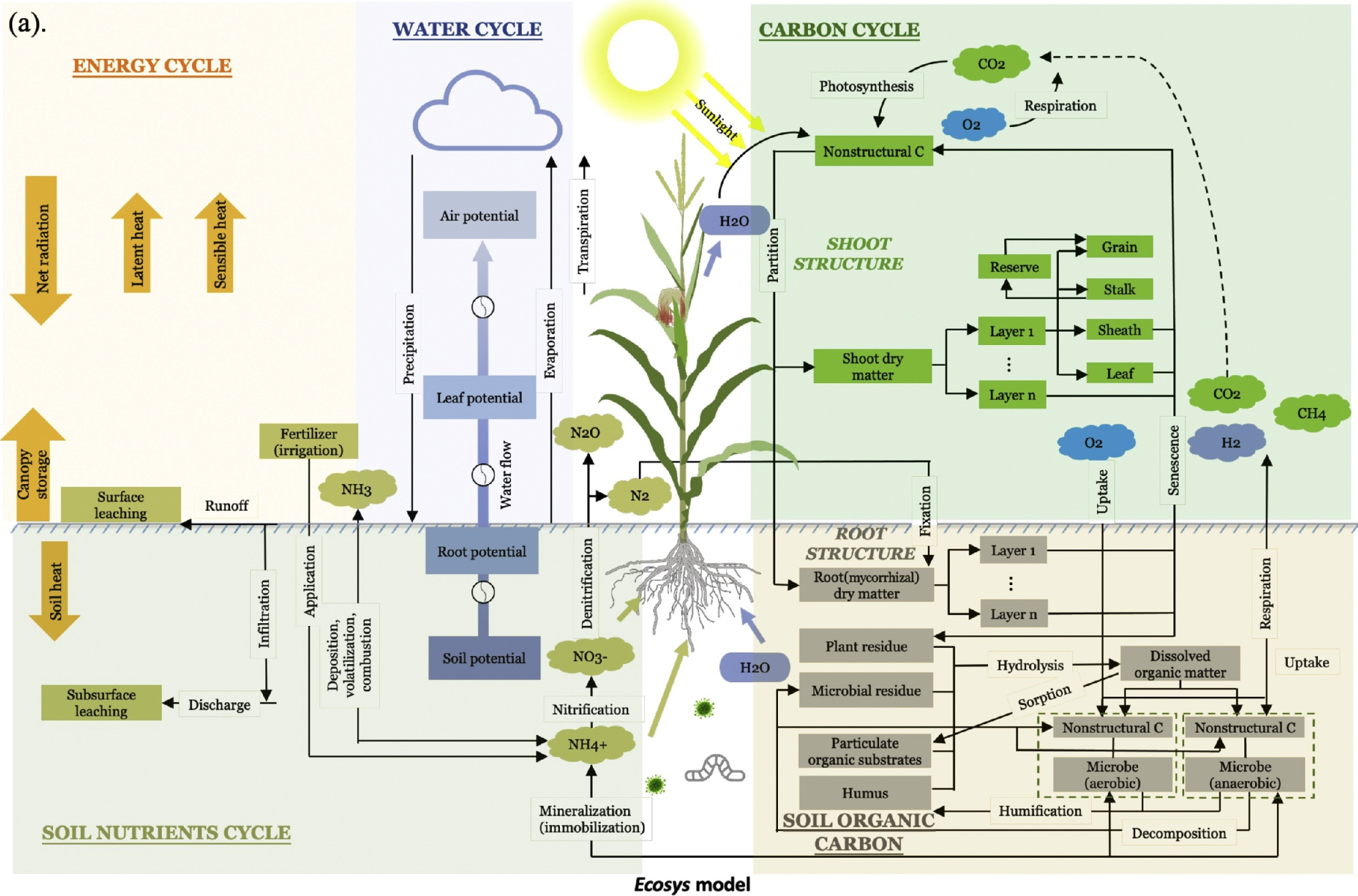
Climate Change Adaptation and Mitigation
We develop models exploring the mechanisms of climate change impacts and then reducing flow-trapping of greenhouse gases into the atmosphere and adjusting to a expected future climate.
1. WinterTurf: A Holistic Approach to Understanding the Mechanisms and Mitigating the Effects of Winter Stress on Turfgrasses in Northern Climates
Winter stresses have long been a challenge for professional turfgrass managers. The long term goal of this project is to better understand turfgrass winter stresses and then identify solutions to this important specialty crop problem. Our approach will include research in plant breeding, genetics, and physiology; efforts to improve resistance to plant disease and thereby reduce pesticide applications; new technological innovations to predict winter stress damage more precisely; and modern approaches to engage and educate stakeholders. The objective that our group is involved will monitor turfgrass stands in cold climates to gain knowledge about the processes that lead to winter damage and how a changing climate will bring new challenges; then use this information to both inform research and create a web-based winter stress injury prediction application. We aim to provide stakeholders with solutions to combat the challenge of winter stresses.
2. DOE: Estimation of Global Methane Soil Sink Using Synthesized Datasets and Knowledge-Guided Machine Learning
The objective of this proposed research is to estimate the spatial and temporal variability in global methane soil sinks using a knowledge- guided machine learning (KGML) framework. This novel framework combines process-based and machine-learning models, and synthesizes multisource direct and indirect measurements of soil methane oxidation to improve model training, interpretability, and accuracy across spatial and temporal scales. Natural methane oxidation by microbes in upland soils is the second largest sink in the global methane budget, but its importance has been widely underestimated. The magnitude and long-term trends of global methane soil sinks are highly uncertain due to overlooked microbial processes and contradicting studies. Accurately quantifying global methane soil sinks is extremely important to reduce biases in current and future global methane budgets.
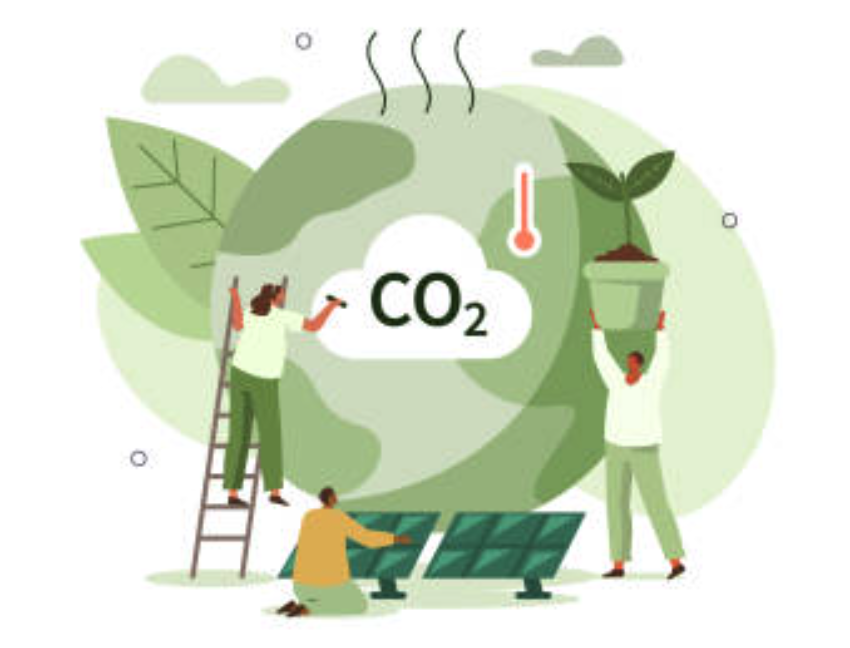
Controlled Environment and Urban Agriculture
As climate change has posed a threat to traditional agriculture, this research aims to use controlled environment to produce high-quality food with optimizing resource-use efficiency. We develop a system to automate farm process, including planting, harvesting, ripeness detection, and yield prediction.
MN Robotics Institute: Robotic Manipulation Towards Fully Autonomous Indoor Farming
Controlled environment agriculture (CEA) such as hydroponics and aeroponics in the greenhouse or vertical farms represents a massive leap in progress toward true agricultural intensification, sustainability, and efficiency. These emerging forms of farming have attracted a surge of interest largely due to their huge potential in boosting productivity. In some well-managed modern greenhouse, reclamation of water, digitally controlled light, nutrients, and microclimate mean that 20 times more yield per acre land can be produced with 90% less water than traditional methods. However, the high labor cost is one of the major barriers for these CEA facilities to be economically feasible. Automation in a cost-effective way is thus the primary demand of this industry. This seed grant supports our pilot efforts on design a robot hand that is capable of planting and harvesting task and fruit ripeness detection and yield prediction.
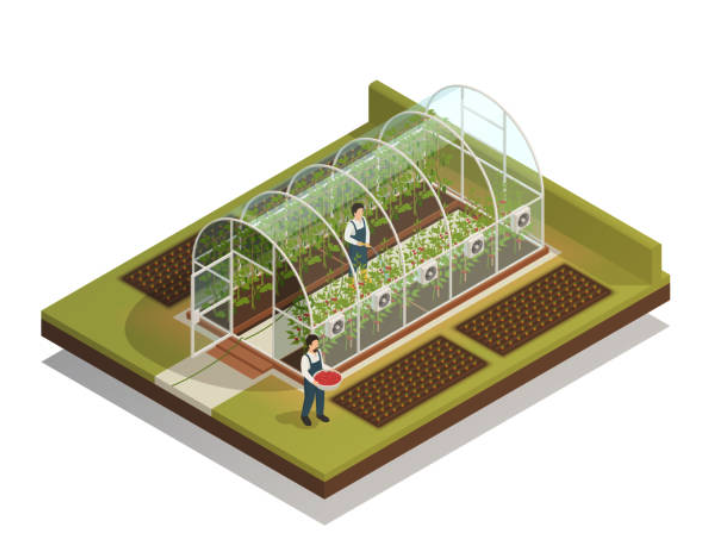